My research is focused on machine learning problems with geometric flavor. The symbiosis between data-driven and model-driven methods opens up new and exciting possibilities to overcome limitations in the new era of machine learning. The data we consume have a unique structure which we can further exploit in learning paradigm used, for example, in computer vision, medical imaging and robotics.
Prof. Dan Raviv
School of Electrical Engineering
ביה"ס להנדסת חשמל
סגל אקדמי בכיר
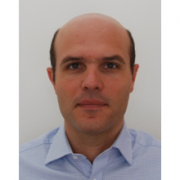
Research:
Education:
Post-doctoral– Massachusetts Institute of Technology (MIT)
Doctorate – Technion, Israel Institute of Technology. Computer Science department.
Master – Technion, Israel Institute of Technology. Computer Science department.
Bachelor – Technion, Israel Institute of Technology. Mathematics department.
Selected publications:
- Affine invariant geometry for non-rigid shapes. D. Raviv and R. Kimmel. International journal of computer vision (IJCV), 111 (1), 2014.
- Scale invariant metrics of volumetric datasets. D. Raviv, and R. Raskar. SIAM Journal on Imaging Sciences, 8(1) (SIIMS), 2015.
- Locally Rigid Averaging (LRA): Expected geometrical mean from stretchable non-rigid observations. D. Raviv, E. Bayro-Corrochano and R. Raskar. International Journal of Computer Vision (IJCV), 2017
- Deep video gesture recognition using illumination invariants. O. Gupta, D. Raviv and R. Raskar. Pattern Recognition, 2018.